AI continues to build the foundation for a remarkable future in
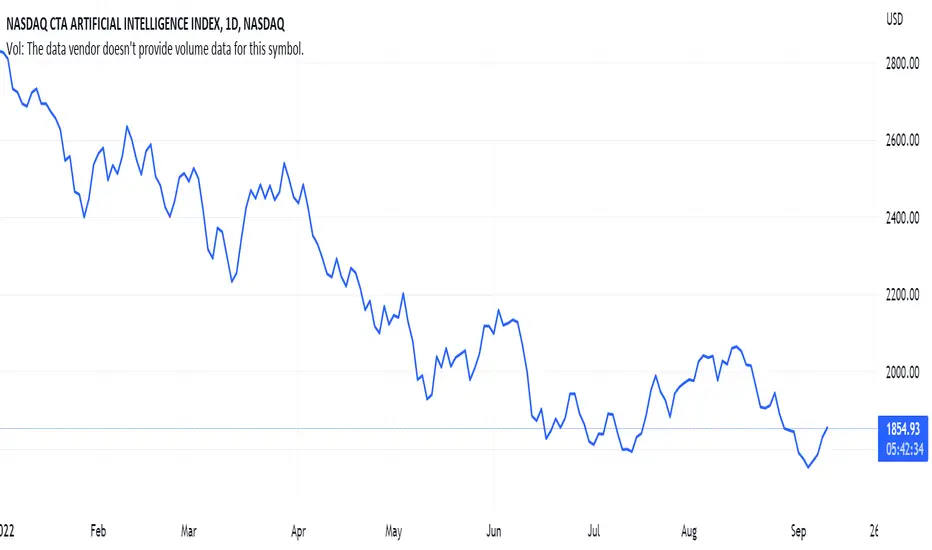
28 July 2022 was an historic day in both biology and artificial intelligence (AI). DeepMind, a firm specialising in AI research owned by Alphabet, made freely available the structural data on more than 200 million proteins from its AlphaFold tool. This represents data on roughly 1 million species and covers the vast majority of known proteins on earth1.
In proteins, shape can determine function
In the late 1990’s into the early 2000’s, the scientific community was awash with news of the race to sequence the human genome. This genome contains the instructions embedded in DNA about how cells should build certain structures, typically through the formation of proteins that are made from different combinations of amino acids.
In a sense, DNA is the instruction manual, amino acids are the building blocks, and proteins are the product. Knowing the code, however, is not the full story.
Looking at figure 1 is instructive on the point. This is the image of a protein that may protect the organism responsible for malaria from an attack by the human immune system. Even if you knew the list and the order of all the amino acids, it would be difficult to go from that list to something that looks like figure 1 in three dimensions.
The importance of the shape of the protein could not be overstated:
It can correspond to the way in which it might react in the presence of different molecules, like those associated with different drug therapies
Variations on the shape—sometimes termed mutations—could be instructive in determining the causal factors of certain symptoms or diseases
Parts of the shape could be used as targets—think of the ‘spike protein’ associated with the virus behind the Covid-19 pandemic, specifically targeted within the mRNA vaccines.
AlphaFold Represents a Leap Forward on the Journey
Scientific breakthroughs are difficult, in that in many cases one builds on another and another and another…a process that can take decades prior to widespread results that impact the lives of the general public. For instance—we sequenced the human genome, but that did not necessarily lead to immediate cures of all sorts of diseases or conditions. mRNA2 research had been occurring for decades, but the Covid-19 pandemic was somewhat of a catalyst to supercharge the process to use it as a case for vaccines.
AlphaFold’s new database is therefore unlikely to lead to immediate cures for difficult conditions. The critical element with regards to how researchers that would have formerly had to undertake a cumbersome process of X-ray crystallography to determine the shape of a given protein could instead go to the database. Experimental techniques would still have their place, but less time would have to be spent on the equivalent of the ‘blank page.’
What’s also incredible is that AlphaFold’s database is, in conjunction with the European Molecular Biology Laboratory’s European Bioinformatics Institute (EMBL-EBI) freely available with a simple interface. It also provides an estimate of the accuracy, recognising that predictions based on AI do not yield perfect results all of the time. Roughly 35% of the 214 million predictions are deemed highly accurate—roughly as good as experimental results. A further 45% are deemed to be accurate enough for many applications3.
Drug discovery—Better therapeutics developed more efficiently
Even before the onset of inflation at the levels we see in the summer of 2022, it was widely recognised that drug development was time consuming and expensive, and as a result many different medications carried with them exorbitant price tags. Any process that could mitigate this pressure without degrading the quality of the therapies would be valuable.
Considering the following could be instructive as the space continues to progress4:
Pipeline growth: 20 smaller companies focused on AI drug discovery, typically with a focus on smaller molecules, over a period from 2010 to 2021, had development pipelines that were roughly 50% as robust as those of 20 of the largest ‘big pharma’ companies. We recognise that the reporting of pipelines may not be perfect and that a molecule in a pipeline is not a finished product, but activity is the first step on the path
Pipeline composition: This is not always disclosed, but from the information available it does indicate that the AI-focused companies will tend to focus on well established biological targets for their therapies, around which much is known. Data is the fuel for AI, and these companies will also want higher chances of success. Bigger pharma companies will be more likely to venture into more emerging areas of drug discovery
Chemical structures and properties: It is too early to be able to draw any robust conclusions regarding AI drug discovery efforts versus big pharma efforts at this point
Discovery Timelines: Preliminary data would appear to indicate that, if traditional approaches would tend to take 5 or 6 years in preclinical phases, AI-focused drug discovery might be able to, in certain cases, take this timeline down to 4 years
We’d note that currently it’s a story of more ‘progress’ than ‘perfection’, in that we would appear to be some distance away from AI being able to fully create new drugs, but AI is representing an entirely new set of tools that could have beneficial impacts. AlphaFold’s database, for example, may provide drug researchers with important inputs and catalysts for different ideas, even if it doesn’t have the immediate answer or cure right there in its system.
Focus on the AI & BioRevolution megatrends
At WisdomTree, we focus on both the AI and the BioRevolution megatrends (click to find out more). What we see here with the case of AlphaFold is an important case study in the fact that AI is a tool that can have the potential to supercharge other megatrends, in this case the BioRevolution. It is no accident that the BioRevolution is ramping up at the same time there are massive amounts of data, massive amounts of processing power and other things like cloud computing readily available. It is very exciting to consider what the coming decades can bring within these areas.
Sources
1 Source: Callaway, Ewen. “’The Entire Protein Universe’: AI Predicts Shape of Nearly Every Known Protein.” Nature. Volume 608. 4 August 2022.
2 mRNA – Messenger ribonucleic acid
3 Source: Callaway, 4 August 2022.
4 Source: Jayatunga et al. “AI in small-molecule drug discovery: a coming wave?” Nature Review: Drug Discovery. Volume 21. March 2022.
This material is prepared by WisdomTree and its affiliates and is not intended to be relied upon as a forecast, research or investment advice, and is not a recommendation, offer or solicitation to buy or sell any securities or to adopt any investment strategy. The opinions expressed are as of the date of production and may change as subsequent conditions vary. The information and opinions contained in this material are derived from proprietary and non-proprietary sources. As such, no warranty of accuracy or reliability is given and no responsibility arising in any other way for errors and omissions (including responsibility to any person by reason of negligence) is accepted by WisdomTree, nor any affiliate, nor any of their officers, employees or agents. Reliance upon information in this material is at the sole discretion of the reader. Past performance is not a reliable indicator of future performance.
In proteins, shape can determine function
In the late 1990’s into the early 2000’s, the scientific community was awash with news of the race to sequence the human genome. This genome contains the instructions embedded in DNA about how cells should build certain structures, typically through the formation of proteins that are made from different combinations of amino acids.
In a sense, DNA is the instruction manual, amino acids are the building blocks, and proteins are the product. Knowing the code, however, is not the full story.
Looking at figure 1 is instructive on the point. This is the image of a protein that may protect the organism responsible for malaria from an attack by the human immune system. Even if you knew the list and the order of all the amino acids, it would be difficult to go from that list to something that looks like figure 1 in three dimensions.
The importance of the shape of the protein could not be overstated:
It can correspond to the way in which it might react in the presence of different molecules, like those associated with different drug therapies
Variations on the shape—sometimes termed mutations—could be instructive in determining the causal factors of certain symptoms or diseases
Parts of the shape could be used as targets—think of the ‘spike protein’ associated with the virus behind the Covid-19 pandemic, specifically targeted within the mRNA vaccines.
AlphaFold Represents a Leap Forward on the Journey
Scientific breakthroughs are difficult, in that in many cases one builds on another and another and another…a process that can take decades prior to widespread results that impact the lives of the general public. For instance—we sequenced the human genome, but that did not necessarily lead to immediate cures of all sorts of diseases or conditions. mRNA2 research had been occurring for decades, but the Covid-19 pandemic was somewhat of a catalyst to supercharge the process to use it as a case for vaccines.
AlphaFold’s new database is therefore unlikely to lead to immediate cures for difficult conditions. The critical element with regards to how researchers that would have formerly had to undertake a cumbersome process of X-ray crystallography to determine the shape of a given protein could instead go to the database. Experimental techniques would still have their place, but less time would have to be spent on the equivalent of the ‘blank page.’
What’s also incredible is that AlphaFold’s database is, in conjunction with the European Molecular Biology Laboratory’s European Bioinformatics Institute (EMBL-EBI) freely available with a simple interface. It also provides an estimate of the accuracy, recognising that predictions based on AI do not yield perfect results all of the time. Roughly 35% of the 214 million predictions are deemed highly accurate—roughly as good as experimental results. A further 45% are deemed to be accurate enough for many applications3.
Drug discovery—Better therapeutics developed more efficiently
Even before the onset of inflation at the levels we see in the summer of 2022, it was widely recognised that drug development was time consuming and expensive, and as a result many different medications carried with them exorbitant price tags. Any process that could mitigate this pressure without degrading the quality of the therapies would be valuable.
Considering the following could be instructive as the space continues to progress4:
Pipeline growth: 20 smaller companies focused on AI drug discovery, typically with a focus on smaller molecules, over a period from 2010 to 2021, had development pipelines that were roughly 50% as robust as those of 20 of the largest ‘big pharma’ companies. We recognise that the reporting of pipelines may not be perfect and that a molecule in a pipeline is not a finished product, but activity is the first step on the path
Pipeline composition: This is not always disclosed, but from the information available it does indicate that the AI-focused companies will tend to focus on well established biological targets for their therapies, around which much is known. Data is the fuel for AI, and these companies will also want higher chances of success. Bigger pharma companies will be more likely to venture into more emerging areas of drug discovery
Chemical structures and properties: It is too early to be able to draw any robust conclusions regarding AI drug discovery efforts versus big pharma efforts at this point
Discovery Timelines: Preliminary data would appear to indicate that, if traditional approaches would tend to take 5 or 6 years in preclinical phases, AI-focused drug discovery might be able to, in certain cases, take this timeline down to 4 years
We’d note that currently it’s a story of more ‘progress’ than ‘perfection’, in that we would appear to be some distance away from AI being able to fully create new drugs, but AI is representing an entirely new set of tools that could have beneficial impacts. AlphaFold’s database, for example, may provide drug researchers with important inputs and catalysts for different ideas, even if it doesn’t have the immediate answer or cure right there in its system.
Focus on the AI & BioRevolution megatrends
At WisdomTree, we focus on both the AI and the BioRevolution megatrends (click to find out more). What we see here with the case of AlphaFold is an important case study in the fact that AI is a tool that can have the potential to supercharge other megatrends, in this case the BioRevolution. It is no accident that the BioRevolution is ramping up at the same time there are massive amounts of data, massive amounts of processing power and other things like cloud computing readily available. It is very exciting to consider what the coming decades can bring within these areas.
Sources
1 Source: Callaway, Ewen. “’The Entire Protein Universe’: AI Predicts Shape of Nearly Every Known Protein.” Nature. Volume 608. 4 August 2022.
2 mRNA – Messenger ribonucleic acid
3 Source: Callaway, 4 August 2022.
4 Source: Jayatunga et al. “AI in small-molecule drug discovery: a coming wave?” Nature Review: Drug Discovery. Volume 21. March 2022.
This material is prepared by WisdomTree and its affiliates and is not intended to be relied upon as a forecast, research or investment advice, and is not a recommendation, offer or solicitation to buy or sell any securities or to adopt any investment strategy. The opinions expressed are as of the date of production and may change as subsequent conditions vary. The information and opinions contained in this material are derived from proprietary and non-proprietary sources. As such, no warranty of accuracy or reliability is given and no responsibility arising in any other way for errors and omissions (including responsibility to any person by reason of negligence) is accepted by WisdomTree, nor any affiliate, nor any of their officers, employees or agents. Reliance upon information in this material is at the sole discretion of the reader. Past performance is not a reliable indicator of future performance.
Pubblicazioni correlate
Declinazione di responsabilità
Le informazioni ed i contenuti pubblicati non costituiscono in alcun modo una sollecitazione ad investire o ad operare nei mercati finanziari. Non sono inoltre fornite o supportate da TradingView. Maggiori dettagli nelle Condizioni d'uso.
Pubblicazioni correlate
Declinazione di responsabilità
Le informazioni ed i contenuti pubblicati non costituiscono in alcun modo una sollecitazione ad investire o ad operare nei mercati finanziari. Non sono inoltre fornite o supportate da TradingView. Maggiori dettagli nelle Condizioni d'uso.